Content from The physics of our analysis
Last updated on 2024-07-30 | Edit this page
Estimated time: 11 minutes
Overview
Questions
- What is the signal we are searching for?
- What are the backgrounds we need to concern ourselves with?
- How will we select the data?
- What is a “boosted jet”?
- What trigger(s) will we be using?
Objectives
- Understand what makes the signal “look” different from our backgrounds?
- How does this translate into how we record and measure particle interactions in CMS?
Introduction
We’re going to reproduce parts of a 2019 CMS study for resonant production of top-antitop pairs. The paper can be found here and hypothesizes a particle with specific properties referred to as \(Z'\) which is produced in our proton-proton collisions and then decays to a top-antitop pair.
\[p p \rightarrow Z' \rightarrow t\overline{t}\]
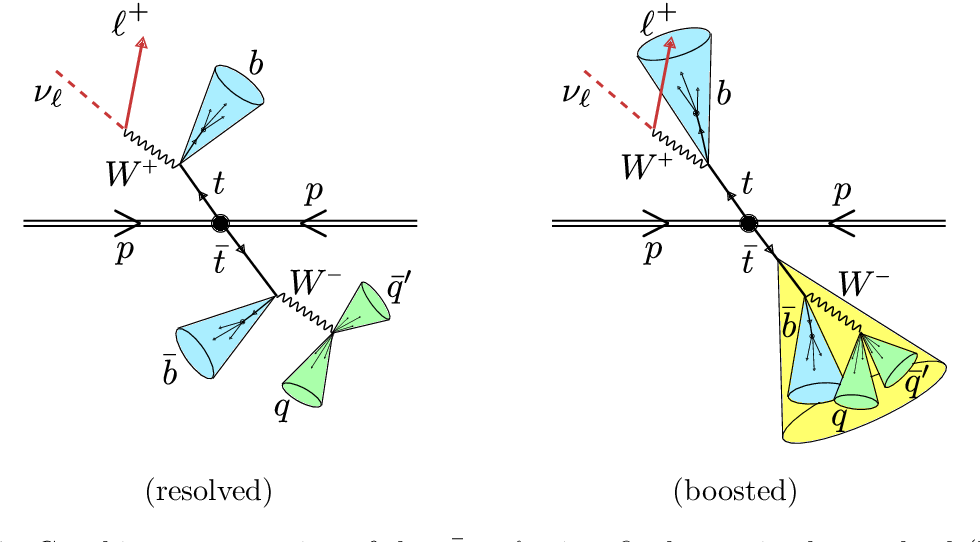
The original analysis searched for the \(Z'\) assuming a range of masses. In the interests of time, we will conduct our search assuming a mass of 2000 GeV/\(c^2\) for the \(Z'\). The Monte Carlo for this process can be found here. It might be helpful to review how to find data and how to understand these records, if you have not done so prior to the lesson.
Selection criteria
A top quark decays almost 100% of the time to a \(b\)-quark and a \(W\) boson. The \(W\) can decay leptonically (to a charged lepton and a neutrino) or hadronically (to a quark and an antiquark). All the quarks hadronize to form jets.
We reconstruct the top quark pair such that one of the top quarks decays leptonically and the other decays hadronically. This is referred to as the semileptonic final state, as opposed to the hadronic final state, when both top quarks decay hadronically, or the leptonic final state, when both top quarks decay leptonically.
We are assuming masses of the \(Z'\) resonance in the TeV range and for this particular search, a mass of 2000 GeV/c\(^2\) (2 TeV/c\(^2\)). The mass of the top quark is 173 GeV/c\(^2\), considerably less than the resonance for which we are searching. This means that a significant amount of the mass-energy of the resonance goes into the momentum of the top quarks, referred to as a boosted final state. In this boosted regime, the individual jets from the hadronically decaying top quark overlap and appear to merge together.
We are going to attempt to calculate the invariant mass of the \(Z'\) by reconstructing it’s 4-momentum from the sum of the 4-momenta of the top quark and antitop quark. Meaning we have four (4) physics “objects” in our final state.
- The jet coming from the \(b\)-quark of the leptonically-decaying top quark.
- The muon coming from the \(W\) of the leptonically-decaying top quark.
- The neutrino coming from the \(W\) of the leptonically-decaying top quark.
- The fully-merged jets of the hadronically-decaying top quark.
Background processes
We will concern ourselves with four (4) physics processes that might mimic our signal and which we identify as possible backgrounds.
- Production of a top-quark pair that decays semileptonically
- Production of a top-quark pair that decays hadronically
- Production of a top-quark pair that decays leptonically
- Production of \(W\) bosons in association with jets and when the \(W\) bosons decay leptonically
Physics requirements
- For the jet from the \(b\) quark,
we are going to make use of the
Jet
physics objects and require that the \(b\)-tagging algorithm is above some threshold and that the jet passes some other combined quality checks. - We will use the
Muon
physics object and require that it is a relatively high momentum muon with \(p_T > 55\) GeV/c and that is is recorded in a fiducial region of \(|\eta| < 2.4\). We will also require that it is isolated from the other jets and that it passes some reconstruction quality thresholds. - For the merged jets, we make use of the
FatJet
object. We will require a \(p_T>500\) GeV/c. While the original analysis tried to tag top quarks by looking at the subjettiness, we now have access to a more sophisticated tagger and so we will use this field and set a threshold to cleanly identify boosted top quark merged jets. - The neutrino will be approximated using the missing energy in the
transverse plane, MET, and we will use the
PuppiMET
object in our calculations. We’ll approximate the mass as 0.
Trigger
We will require that events pass the HLT_TkMu50
trigger:
the high-level trigger that requires a track consistent with a muon with
\(p_T > 50\) GeV/c.
Luminosity masking
In a previous lesson, you learned that the luminosity is calculated for “good” runs and so we need to make sure we only use those runs when we process collision data.
We’ll be downloading and using this file to match and select these good runs.
Key Points
- We reconstruct the final state in the semileptonic decay of the top-quark pair
- The high mass assumption of the \(Z'\) resonance leads to boosted final states
- We can make use of specific reconstruction algorithms to identify these final states
Content from Write the code
Last updated on 2024-07-30 | Edit this page
Estimated time: 32 minutes
Overview
Questions
- How do you write the code that matches our physics selection criteria?
- How do we keep track of everything?
- What do we write out when we process a file?
Objectives
- Learn to use
awkward
arrays to select subsets of the data - Understand how to apply the luminosity mask
- Make a first-order plot of some of our variables of interest
- Process at least one file of both simulation and collision data
Introduction
There is a significant amount of code to run here and so we have written the majority of it in a Jupyter notebook. You can run most of the code on its own, but you should take the time to read and understand what is happening. In some places, you need to modify the code to get it to run.
First, start your python docker container, following the lessons from
the
pre-exercises. I am on a Linux machine, and I have already created
the cms_open_data_python
directory. So I will do the
following
Start the container with
BASH
docker run -it --name my_python -P -p 8888:8888 -v ${workpath}/cms_open_data_python:/code gitlab-registry.cern.ch/cms-cloud/python-vnc:python3.10.5
You will get a container prompt similar this:
OUTPUT
cmsusr@4fa5ac484d6f:/code$
Before we start our Jupyter environment, let’s download the notebook we’ll be using with the following command.
BASH
wget https://raw.githubusercontent.com/cms-opendata-workshop/workshop2024-lesson-event-selection/main/instructors/data_selection_lesson.ipynb
Now I will start Jupyter lab as follows.
and open the link that is printed out in the message.
How to follow this lesson
While some of the code will be explained on this web page, the majority of the code and explanations of the code are written out in the Jupyter notebook. Therefore, you should primarily following along there.
I will use this webpage for the lesson to provide guideposts and checkpoints that we can refer to as we work through the lesson.
Running through the selection steps (in the Jupyter notebook)
Preparing the environment
We will be making extensive use of the uproot
and
awkward
python libraries, the pandas
data
library, and a few other standard python libraries. The first part of
the notebook Install and upgrade libraries asks you to
do just that, in order to ensure a consistent environment.
Depending on your connection, it should take 1-2 minutes to upload and import the libraries.
We’ve also prepared some helper code that makes it easier to work with the data in this lesson. You can see the code here but we will explain the functions and data objects in this notebook.
Read in some files
Run through the notebook for the Download essential files section.
How many input NanoAOD files will we process?
How many collision files are there? How many signal files are there? How many files are there combined in the background sample files?
You will run
And get
OUTPUT
68 FILE_LIST_Wjets.txt
152 FILE_LIST_collision.txt
4 FILE_LIST_signal_M2000.txt
146 FILE_LIST_tthadronic.txt
49 FILE_LIST_ttleptonic.txt
138 FILE_LIST_ttsemilep.txt
557 total
So there are 152 files in the collision
dataset.
4 files in our signal_M2000
dataset.
If we add up the background samples of ttXXX
and
Wjets
we find there are 401 total files.
Apply the cuts
Run through the next sections in the notebook to set up the cuts.
Check your understanding as you go.
Reconstruct the resonance candidate mass
To reconstruct the \(z'\) candidate mass in a computationally-efficient way, we are going to make use of the Vector library, which works very well with awkward, as you can see in these examples.
It allows for very simple code that will automatically calculate all combinations of particles when reconstructing some parent candidate.
Plot
If you are able to run though Use the cuts and calculate some values, you should have been able to produce a basic plot.
Plot the ttbar mass
Key Points
- Awkward arrays allow for a simplified syntax when making cuts to select the data
- You need to be careful to distinguish between cuts on events and cuts on particles
Content from Putting it all together
Last updated on 2024-07-30 | Edit this page
Estimated time: 22 minutes
Overview
Questions
- Do we process collision data differently from our simulation data?
- How might we process multiple files?
Objectives
- Run over multiple collision or simulation files
- Develop a sense of how long it takes to run over lots of data and how you might scale it up
Introduction
We’re going to continue to work with the same Jupyter notebook as before, picking up exactly where we left off with the Processing data files section.
Processing data
Follow the notebook to develop a sense of how we apply the luminosity masking, following the concepts introduced in a previous lesson.
Processing multiple files
The notebook has an example of how all of the previous cuts and data processing can be put into one function that is easily called.
You can return the data in any format you like, but we chose to use pandas DataFrame objects. They are widely used in many data science communities and can make simple data analysis much easier.
Make sure you get through the examples so that you have processed at least a few files from different datasets.
How much data should I run over?
It can take 6-12 hours to run over all the data for this lesson, depending on your computer’s speed and your internet connection.
We recommend that for this lesson you do not process all the data yourself. For future lessons, we will have processed all the data for you.
Activity!
Once you are able to run over multiple files, try to make some additional plots, either from the dataframes or from the earlier code where you are processing things step-by-step.
Try making some plots to compare the signal with one of the background samples. Are they different? Similar?
Are either of them similar to the collision data?
Add your plots to the Google document listed in the Mattermost channel for this lesson.
Key Points
- It it up to you how you want to save your reduced data and how to keep track of everything
- There are many options, but think about how you might scale things up