Content from Introduction
Last updated on 2024-07-28 | Edit this page
Estimated time: 90 minutes
Overview
Questions
- Why is collaboration and knowledge sharing important in particle physics?
- What is the significance of open data and the open science community?
- What direction and challenges will we take in this hackathon?
Objectives
- Understand the importance of collaboration and knowledge sharing in particle physics.
- Learn about the significance of open data and the role of the open science community.
- Get an overview of the different lessons and challenges in the hackathon.
Welcome to the CMS Open Data Workshop & Hackathon 2024!
Importance of Collaboration and Knowledge Sharing
In the field of particle physics, collaboration and knowledge sharing are crucial. The CMS Open Data Workshop & Hackathon aims to foster these values by providing a platform for participants to work together, share insights, and learn from one another. By collaborating on complex problems and sharing our findings, we can push the boundaries of what we know and achieve breakthroughs that would be impossible to accomplish alone.
Testimonial
By working together, we can leverage our collective expertise and creativity to solve complex problems and advance the field of particle physics.
The Importance of Open Data and the Open Science Community
Open data is a cornerstone of modern scientific research. By making data freely available to the public, we enable a broader range of scientists and enthusiasts to engage with it, leading to more robust and innovative discoveries. The open science community thrives on transparency, accessibility, and collaboration, and CMS Open Data is a perfect example of these principles in action. Through this hackathon, we aim to demonstrate the power of open data and encourage more people to contribute to the open science movement.
Testimonial
Open data allows for greater transparency and reproducibility in research, fostering innovation and enabling more people to contribute to scientific discoveries.
Direction and Challenges of the Hackathon
This section is tailored for our remote participants who do not have an active research task for Open Data. These activities are designed to provide a comprehensive learning experience and allow you to engage deeply with particle physics, data analysis, and machine learning from a distance.
Key Activities
- Particle Physics Playground: Dive into fundamental concepts in particle physics through interactive exercises. Revisit the Particle Physics Primer pre-learning lesson to explore basic principles and engage with various scenarios to enhance your understanding.
- Particle Discovery Lab: Analyze real particle collision data from the CMS experiment using Python containers. Clone repositories, follow instructions, and perform both basic and advanced data analysis tasks to identify different particles and gain hands-on experience with real-world data.
- Machine Learning 1: Get introduced to the application of machine learning in high-energy physics (HEP). Learn initial concepts and practical applications of ML techniques in analyzing particle collision data.
- Machine Learning 2: Delve deeper into supervised and unsupervised learning methods. Follow detailed instructions to apply these techniques for classifying particle collisions, and gain practical experience in data preparation, model training, and evaluation.
- Analysis Grand Challenge: Participate in a more extensive exercise using up-to-date HEP software tools. Engage with tasks such as creating synthetic data from older CMS data, tackling generative modeling challenges, and validating complex models.
These activities are designed to provide a rich learning experience and help you contribute meaningfully to the open science community. Enjoy exploring and collaborating with fellow participants throughout the hackathon!
Challenge Yourself and Collaborate!
Join us in this exciting journey of discovery and innovation. By participating in the CMS Open Data Workshop & Hackathon, you are contributing to a global community of scientists and enthusiasts working towards a common goal. Let’s push the boundaries of our knowledge together!
Key Points
- Collaboration and knowledge sharing are essential in advancing particle physics research.
- Open data enables greater transparency, accessibility, and innovation in scientific research.
- This hackathon offers a variety of lessons and challenges suitable for participants with different interests and skill levels.
Content from Particle Physics Playground
Last updated on 2024-07-28 | Edit this page
Estimated time: 5 minutes
Overview
Questions
- What are the fundamental concepts in particle physics?
- How can we analyze particle decay patterns?
- What tools are available for practical analysis of particle physics data?
Objectives
- Gain a solid understanding of fundamental particle physics concepts.
- Learn how to analyze particle decay patterns.
- Familiarize yourself with tools for practical analysis of particle physics data.
Particle Physics Playground
This activity offers a variety of exercises designed to help undergraduates understand fundamental concepts in particle physics through practical analysis of CMS Open Data.
Overview
The Particle Physics Playground provides an engaging and interactive way for participants to delve into the core principles of particle physics. By working with real CMS Open Data, students will enhance their theoretical knowledge through hands-on experience.
Discussion forums
Make sure to join the Mattermost channel for this activity to engage directly with the workshop instructors and fellow participants.
Pre-learning Lesson
Participants are encouraged to review the Particle Physics Primer pre-learning lesson. This foundational lesson is equipped with lectures and exercises covering the following topics:
Fundamental Concepts
Participants will explore key concepts in particle physics, such as the Standard Model, particle interactions, and conservation laws. Understanding these principles is crucial for analyzing and interpreting particle collision data.
Analyzing Particle Decay Patterns
One of the main tasks in this lesson is to analyze particle decay patterns. By studying the way particles decay, participants can learn about the properties of different particles and the forces that govern their interactions. This includes identifying decay channels, measuring lifetimes, and calculating branching ratios.
Practical Analysis Tools
Participants will be introduced to various tools and techniques used in particle physics analysis. This includes the use of Python and Jupyter notebooks hosted on Google Colab for data analysis, as well as simplified data formats provided by the Particle Physics Playground website. The goal is to equip students with the practical skills needed to conduct their own analyses and contribute to ongoing research.
Recommendations
Activity Suggestions
- Watch the Lectures: Start by watching the first three lectures by Dr. Allison Hall to build a strong theoretical foundation.
- Try the Exercises: Attempt the associated exercises to test your understanding and apply what you’ve learned.
-
Explore the Toy Analyses: Engage with the toy
analyses to gain hands-on experience with real CMS data, focusing on:
- Working with Colab and understanding data formats.
- Learning about particle lifetimes and identifying detectable particles.
- Calculating masses using 4-vectors and creating histograms.
- Discovering new particles by analyzing decay products.
- Discuss and Collaborate: Use discussion forums (Mattermost channel) or collaborative platforms to share your findings, ask questions, and work with peers.
Additional Resources
- Particle Physics Primer Videos: Watch public-oriented videos for a broad overview.
- Standard Model References: Review materials on the Standard Model and its historical development.
- Advanced Lectures: For those interested, watch the remaining lectures covering neutrino physics and dark matter.
You Have Choices!
While Python and Jupyter notebooks are the primary tools for this activity, feel free to explore other tools and file formats that suit your needs. The goal is to learn and apply particle physics analysis techniques in a way that works best for you.
Key Points
- Fundamental concepts in particle physics.
- Techniques for analyzing particle decay patterns.
- Practical tools and techniques for particle physics analysis.
Content from Particle Discovery Lab
Last updated on 2024-07-28 | Edit this page
Estimated time: 90 minutes
Overview
Questions
- How can we identify different particles in collision data?
- What are the characteristics of muons in the dataset?
- How do we perform basic and advanced data analysis in particle physics?
Objectives
- Reconstruct decays of an unknown particle X to 2 muons.
- Use histograms to display the calculated mass of particle X.
- Learn to fit and subtract background contributions from data.
- Understand uncertainty propagation throughout the analysis.
- Identify the discovered particle and compare its properties to known values.
Particle Discovery Lab
The goal of the Particle Discovery Lab, is to reconstruct decays of an unknown particle X (initial state) to 2 muons (final state). Participants will display histograms for the calculated mass of particle X and learn about fitting and subtracting background distributions from data.
Uncertainty propagation concepts are included at each step of the analysis. After isolating the signal distribution, participants will determine which particle they have discovered and compare its properties (mass and width) to known values.
Get Ready
-
Prepare Your Environment:
- Ensure that the
my_python
container is ready. Refer to the pre-exercise instructions for Docker setup and container creation.
- Ensure that the
-
Set Up and Launch Jupyter Lab:
-
Execute the following commands in your terminal:
After running the last command, open the provided link in your browser to access Jupyter Lab.
-
-
Verify Files:
- Ensure that the following files are present in the
Particle_Discovery_Lab
directory after cloning the repository:pollsf.py
Particle_Discovery_Lab.ipynb
DoubleMuParked_100K.pkl
- Ensure that the following files are present in the
Instructions for the Exercise
-
Launch Jupyter Lab:
- In Jupyter Lab, navigate to
Particle_Discovery_Lab.ipynb
from the file menu and open it.
- In Jupyter Lab, navigate to
-
Complete the Notebook:
- Follow the instructions in the notebook to complete the analysis interactively. You will perform tasks such as plotting histograms, fitting data, and analyzing uncertainties.
Discussion forums
Make sure to join the Mattermost channel for this activity to engage directly with the workshop instructors and fellow participants.
Visualize with CMS Spy WebGL
To enhance your understanding and visualization of the particle collision events, use the CMS Spy WebGL visualizer. This tool provides a 3D visualization of the CMS collision data, allowing you to better grasp the spatial distribution and interactions of particles.
Recommendations for Hackathon Activities
Participants in the hackathon can leverage their skills and the themes explored in the Particle Discovery Lab to tackle innovative challenges and projects. Here are some suggested activities:
- Advanced Particle Identification Algorithms: Develop and implement advanced algorithms for particle identification using collision data.
- Enhanced Data Visualization Tools: Create interactive tools for exploring and analyzing CMS collision data in real-time.
- Integration of Machine Learning: Apply machine learning techniques to automate data analysis and improve particle identification accuracy.
- Collaborative Analysis Projects: Form teams to tackle complex analysis challenges or develop new methodologies for studying particle interactions.
- Educational Outreach and Visualization: Design educational materials or demos that explain particle physics principles using CMS collision data.
- Open Data Innovation: Develop tools or platforms to enhance accessibility and usability of CMS Open Data for the scientific community.
These activities encourage innovation, collaboration, and exploration of particle physics concepts beyond the basic lab exercises.
Key Points
- Introduction to particle collision data.
- Techniques for identifying particles such as muons and electrons.
- Methods for performing both basic and advanced data analysis.
Content from Introduction to Machine Learning in HEP
Last updated on 2024-07-28 | Edit this page
Estimated time: 5 minutes
Overview
Questions
- How can machine learning be applied to particle physics data?
- What are the steps involved in preparing data for machine learning analysis?
- How do we train and evaluate a machine learning model in this context?
Objectives
- Learn the basics of machine learning and its applications in particle physics.
- Understand the process of preparing data for machine learning.
- Gain practical experience in training and evaluating a machine learning model.
Overview
Machine learning (ML) is a powerful tool for extracting insights from complex datasets, making it invaluable in high-energy physics (HEP) research. The Machine Learning in High-Energy Physics (HEP) activity bridges the gap between data science and particle physics, utilizing CMS Open Data to explore real-world applications. Participants will learn to leverage ML algorithms to analyze particle collision data, enabling them to classify events, discover new particles, and enhance their understanding of fundamental physics.
Prerequisites
Before diving into ML in HEP, participants should have a basic understanding of: - Programming fundamentals (Python recommended) - Data handling and visualization - Elementary statistical concepts (mean, variance, etc.)
Discussion forums
Make sure to join the Mattermost channel for this activity to engage directly with the workshop instructors and fellow participants.
Let’s get the basics clear
Machine learning (ML) is a branch of artificial intelligence (AI) and computer science that focuses on the using data and algorithms to enable AI to imitate the way that humans learn, gradually improving its accuracy. If that is not clear, please watch this video.
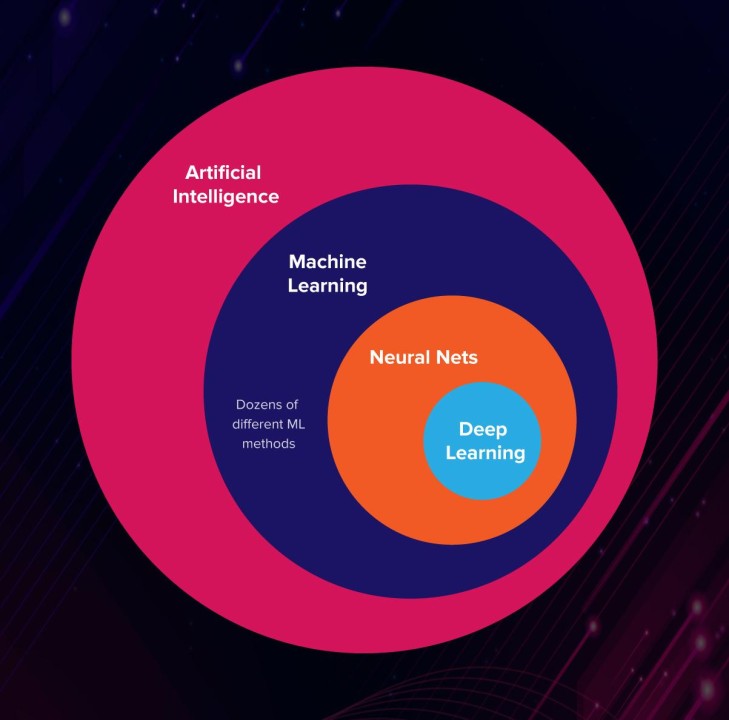
Callout
Machine learning, deep learning, and neural networks are all sub-fields of artificial intelligence. However, neural networks is actually a sub-field of machine learning, and deep learning is a sub-field of neural networks.
To have an overview of neural networks, visit 3Blue1Brown’s basics of neural networks, and the math behind how they learn.
Data Acquisition and Understanding
By now we must have a basic understanding of how Machine Learning functions, to use this in the realm of High Energy Physics, we must have the following basics.
CMS Open Data Overview: - Accessing and understanding the CMS Open Data repository. - Types of datasets available (e.g., AOD, MiniAOD, NanoAOD) and their differences. - Introduction to the CMS experiment and its detectors.
Data Preparation - Cleaning and Preprocessing
As you dive into the hackathon, keep in mind that feature engineering—like selecting relevant features, creating new ones to enhance model performance, and using dimensionality reduction techniques play a crucial role in both supervised and unsupervised learning. Mastering these techniques will significantly impact your models’ ability to learn from and make sense of your data, so be sure to leverage them effectively in your projects!
- Handling missing data points and outliers.
- Normalizing data to ensure consistency across features.
- Exploratory data analysis (EDA) to understand distributions and correlations.
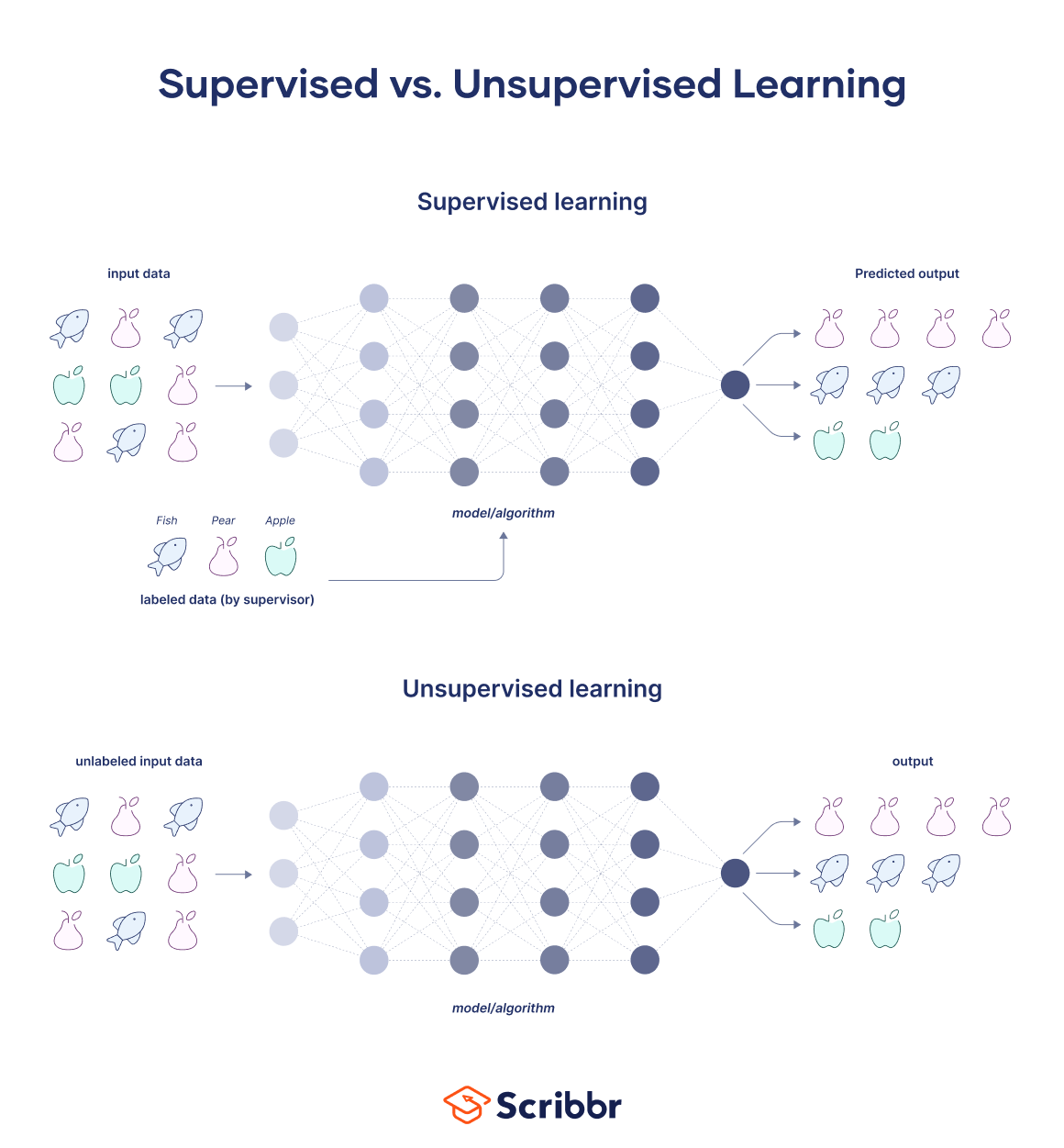
You can get a glimpse of the differences in this video.
Supervised Learning in HEP
Basics of Supervised Learning
- Understanding labeled datasets and target variables.
- Classification tasks: distinguishing particle types (e.g., muons, electrons).
- Regression tasks: a possible application in HEP can be predicting particle properties (e.g., energy, momentum).
Model Selection and Training
- Choosing appropriate algorithms (e.g., Decision Trees, Random Forests, Neural Networks).
- Cross-validation techniques to optimize model performance.
- Hyperparameter tuning to fine-tune model behavior.
Model Evaluation
- Metrics: accuracy, precision, recall, F1-score.
- Confusion matrices and ROC curves for performance visualization.
- Interpreting results and refining models based on feedback: Watch this video for Learning Curves In Machine Learning explanation.
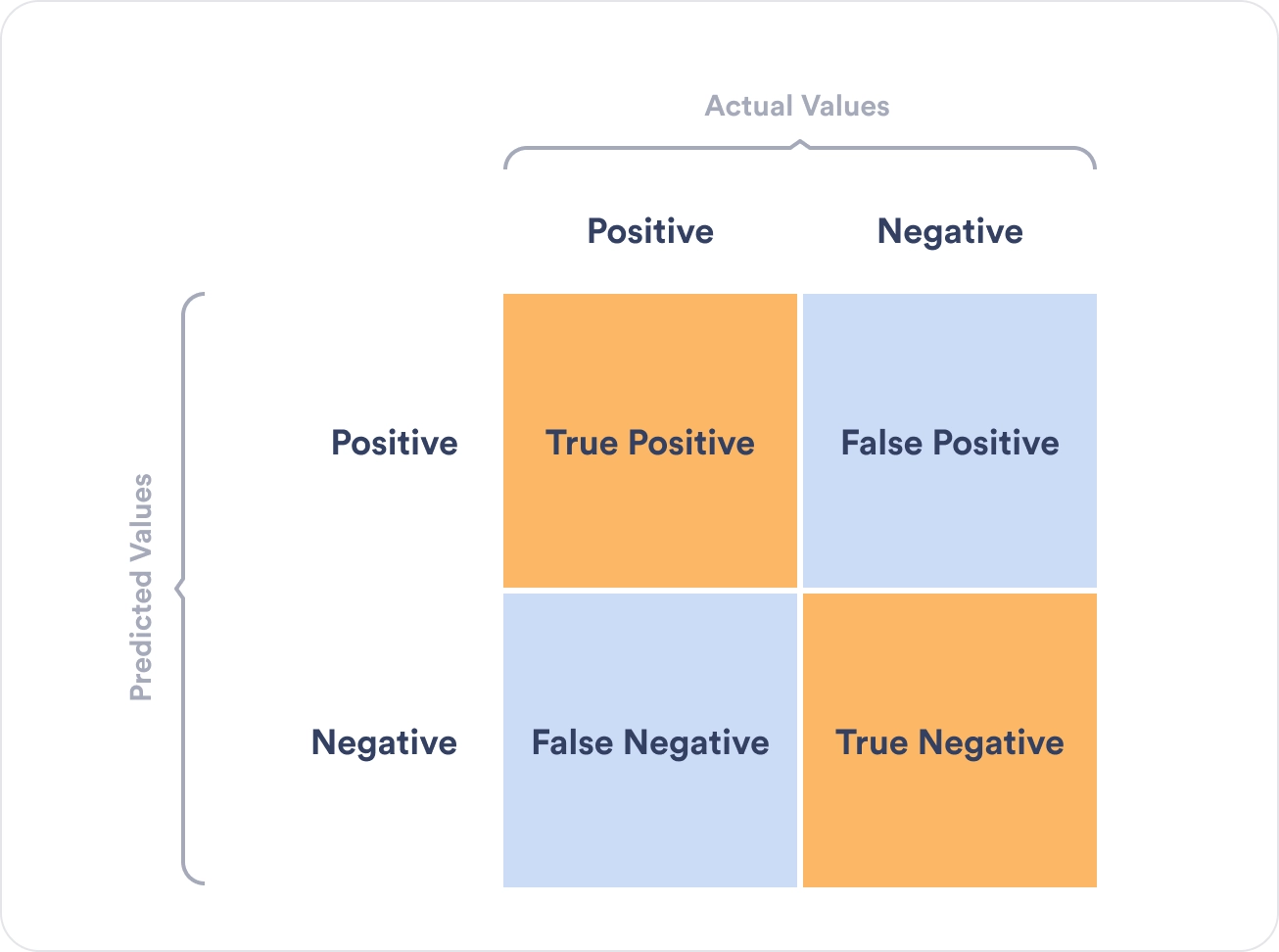
Unsupervised Learning in HEP
Basics of Unsupervised Learning
- Clustering algorithms (K-means, DBSCAN) for grouping similar events.
- Anomaly detection techniques to identify unusual data points.
- Dimensionality reduction methods (PCA, LDA) for visualizing high-dimensional data.
Conclusion
The Machine Learning with Open Data lesson equips participants with fundamental skills to apply ML techniques effectively in high-energy physics research. By mastering data preparation, model training, and evaluation, participants gain insights into both machine learning principles and their practical applications in particle physics.
Key Points
- Introduction to machine learning in particle physics.
- Comprehensive data preparation for machine learning analysis.
- Supervised and unsupervised learning techniques specific to HEP.
- Advanced ML applications in particle physics research.
Content from Machine Learning Practical Applications
Last updated on 2024-07-31 | Edit this page
Estimated time: 5 minutes
Overview
Questions
- How can machine learning be applied to particle physics data?
- What are the steps involved in preparing data for machine learning analysis?
- How do we train and evaluate a machine learning model in this context?
Objectives
- Learn the basics of machine learning and its applications in particle physics.
- Understand the process of preparing data for machine learning.
- Gain practical experience in training and evaluating a machine learning model.
Practical Application of Machine Learning in Particle Physics
Machine learning techniques, such as Convolutional Neural Networks (CNNs) and autoencoders, play pivotal roles in analyzing particle physics data. This section provides insights into their architectures, training processes, and practical applications within the field.
Discussion forums
Make sure to join the Mattermost channel for this activity to engage directly with the workshop instructors and fellow participants.
Supervised Learning - Convolutional Neural Networks (CNNs)
Purpose and Architecture
CNNs are specialized neural networks designed for processing grid-like data, such as images. In particle physics, CNNs are instrumental in tasks requiring image classification, object detection, and image segmentation:
- Purpose: CNNs excel in supervised learning scenarios where labeled data is available for training.
- Architecture: They comprise convolutional layers that extract features hierarchically, pooling layers for spatial dimension reduction, and dense layers for final classification.
- Training: CNNs learn through backpropagation, adjusting weights to minimize classification error or regression loss.
- Applications: In particle physics, CNNs are used to classify particle types, analyze detector images for anomalies, and segment regions of interest in collision data.
Practical Example
This project explores the application of deep learning techniques in
high-energy physics using data from the CMS experiment at the LHC. The
repository includes analyzers, scripts, and notebooks to process
collision data and train convolutional neural networks (CNNs) for
particle classification. By transforming collision data into images and
using various CNN architectures, the project aims to classify
high-energy particle collision outcomes with high accuracy. The
poet_realdata.py
script and
MuonAnalyzer_realdata.cc
analyzer are based on the original
configuration and analyzers used in the final version of the CMS
Open Data Workshop 2022, ensuring consistency and relevance of the
data analysis techniques and tools employed in this project with those
taught during the workshop.
Credit
This section and the associated project was developed by José David Ochoa Flores, Daniela Merizalde, Xavier Tintin, Edgar Carrera, David Mena, and Diana Martinez, a collaborative research project in data science and particle physics from Universidad San Francisco de Quito and Escuela Politéncica Nacional.
Link to repo: GitHub
Link to paper: Springer Link
Unsupervised Learning - Autoencoders
Purpose and Architecture
Autoencoders are unsupervised learning models that learn efficient data representations without explicit supervision. They are versatile in particle physics for tasks such as dimensionality reduction, anomaly detection, and feature extraction:
- Purpose: Autoencoders are adept at learning from unlabeled data to capture underlying patterns or compress data representations.
- Architecture: They consist of an encoder network to compress input into a latent space and a decoder network to reconstruct the input from this representation.
- Training: Autoencoders minimize reconstruction error during training, optimizing parameters to improve data reconstruction quality.
- Applications: In particle physics, autoencoders are used to denoise detector data, detect rare events or anomalies in experimental data, and extract meaningful features for subsequent analysis.
Practical Example
The QCD School 2024 ML project is an educational initiative designed to introduce participants to the application of machine learning in high energy physics, specifically through anomaly detection using unsupervised learning. The project provides a hands-on tutorial for designing and implementing a tiny autoencoder (AE) model, which is trained to identify potentially new physics events from proton collision data obtained from the CMS Open Data. In this example you will learn to compress and decompress data using the autoencoder, train it on background data, and evaluate its performance on both background and New Physics simulated samples. The project also covers advanced techniques like quantization-aware training using QKeras and model deployment on FPGA firmware with hls4ml, providing a comprehensive learning experience that bridges theoretical concepts with practical implementation.
Credit
This section and the QCD School 2024 ML project were developed by Thea Klaeboe Aarrestad, a particle physicist at ETH Zurich specializing in real-time AI and FPGA inference in the CMS experiment at CERN.
Link to repo: GitHub
For this workshop, you can perform this exercise in a slightly newer python container:
BASH
export workpath=$PWD
mkdir cms_open_data_ML
chmod -R 777 cms_open_data_ML
docker run -it --name my_ML -P -p 8888:8888 -v ${workpath}/cms_open_data_ML:/code gitlab-registry.cern.ch/cms-cloud/python-vnc:python3.10.12
code/$ pip install qkeras==0.9.0 tensorflow==2.11.1 hls4ml h5py mplhep cernopendata-client pydot graphviz
code/$ pip install --upgrade matplotlib
code/$ pip install fsspec-xrootd
Key Differences
- Supervised vs. Unsupervised: CNNs require labeled data for training (supervised), while autoencoders learn from unlabeled data (unsupervised).
- Output: CNNs produce predictions based on input data labels (classification/regression), whereas autoencoders reconstruct input data or learn compressed representations.
- Use Cases: CNNs are suitable for tasks requiring precise classification or segmentation in structured data like detector images. Autoencoders excel in exploratory tasks, anomaly detection, and dimensionality reduction in complex datasets.
Practical Considerations
Understanding these machine learning techniques equips researchers with powerful tools to analyze CMS Open Data effectively. By mastering CNNs and autoencoders, participants can enhance their ability to derive insights, classify particles, and uncover new physics phenomena from particle collision data.
Key Points
- Supervised vs. Unsupervised: CNNs require labeled data for training, making them suited for supervised learning tasks where the model learns from explicit examples with known outcomes. Autoencoders, in contrast, utilize unlabeled data and excel in unsupervised learning, focusing on learning data representations and detecting anomalies without predefined labels.
- Output: CNNs produce predictions or classifications based on input data labels (classification/regression), whereas autoencoders aim to reconstruct input data or generate compressed representations for further analysis.
- Use Cases: CNNs are ideal for tasks involving structured data such as detector images, where precise classification or segmentation is needed. Autoencoders are particularly useful for exploratory tasks, anomaly detection, and dimensionality reduction in complex datasets where direct supervision is not available.
Content from Analysis Grand Challenge
Last updated on 2024-07-30 | Edit this page
Estimated time: 5 minutes
Overview
Questions
- How do we perform a cross-section measurement with CMS Open Data?
- What are the challenges of processing large datasets in particle physics?
- How do we ensure reproducibility and scalability in analysis workflows?
Objectives
- Perform a cross-section measurement using CMS Open Data.
- Understand the challenges and methods for processing large particle physics datasets.
- Gain experience in creating reproducible and scalable analysis workflows.
Analysis Grand Challenge
This project is designed for more experienced programmers looking for a challenging activity using CMS Open Data.
Overview
The Analysis Grand Challenge (AGC) is aimed at participants who have a solid programming background and are eager to tackle complex problems. This challenge involves performing the final steps in an analysis pipeline at scale to test workflows envisioned for the High-Luminosity Large Hadron Collider (HL-LHC).
Setup for Open Data workshop participants
You will need a slightly newer python container to install all the packages needed for this challenge
export workpath=$PWD
mkdir cms_open_data_AGC
chmod -R 777 cms_open_data_AGC
docker run -it --name my_agc -P -p 8888:8888 -v ${workpath}/cms_open_data_AGC:/code gitlab-registry.cern.ch/cms-cloud/python-vnc:python3.10.12
The AGC \(t\bar{t}\) analysis repository contains a file of requirements. Download this file and install the requirements:
## alternate to wget: git clone https://github.com/iris-hep/analysis-grand-challenge
code/$ wget https://raw.githubusercontent.com/iris-hep/analysis-grand-challenge/main/analyses/cms-open-data-ttbar/requirements.txt
code/$ pip install -r requirements.txt
code/$ pip uninstall xgboost scikit-learn
code/$ pip install scikit-learn xgboost
Notes for changes needed by workshop participants
- When you come to this line in the notebook: https://github.com/iris-hep/analysis-grand-challenge/blob/main/analyses/cms-open-data-ttbar/ttbar_analysis_pipeline.py#L514,
Change
af=utils.config["global"]["AF"]
toaf='local'
- more TBA…
Analysis Pipeline
Participants will work on a cross-section measurement using 2015 CMS Open Data. This includes:
- Columnar Data Extraction: Extracting data from large datasets in a columnar format.
- Data Processing: Filtering events, constructing observables, and evaluating systematic uncertainties.
- Histogramming: Summarizing the processed data into histograms.
- Statistical Model Construction: Building statistical models based on the histograms.
- Statistical Inference: Performing statistical analysis to infer the cross-section measurement.
- Visualization: Creating relevant visualizations for each step of the analysis.
Working with CMS Open Data
Using older CMS data presents unique challenges, such as analyzing data formats within the CMSSW software framework. Participants will learn strategies for overcoming these obstacles, ensuring their analyses are robust and accurate even when working with older CMS Open Data.
Discussion forums
Make sure to join the Mattermost channel for this activity to engage directly with the workshop instructors and fellow participants.
Reproducibility and Scalability
An essential aspect of the AGC is ensuring that the analysis workflow is reproducible and can scale to the requirements of the HL-LHC. Participants will learn how to:
- Utilize tools and services for data access and event selection (e.g., Rucio, ServiceX).
- Implement histogramming and summary statistics using tools like Coffea and cabinetry.
- Construct and fit statistical models using software like Pyhf.
- Capture the entire analysis workflow for future reinterpretation using standards and services like REANA and RECAST.
Practical Application
In this project, participants will apply their knowledge to perform a cross-section measurement. This involves building and executing the analysis pipeline, rigorously validating the results, and ensuring the workflow is reproducible and scalable.
For more detailed information and guidelines, please refer to the Analysis Grand Challenge Documentation.
Key Points
- Cross-section measurement using CMS Open Data.
- Challenges and methods for processing large datasets.
- Creating reproducible and scalable analysis workflows.